
Original Link: https://www.anandtech.com/show/15578/cloud-clash-amazon-graviton2-arm-against-intel-and-amd
Amazon's Arm-based Graviton2 Against AMD and Intel: Comparing Cloud Compute
by Andrei Frumusanu on March 10, 2020 8:30 AM EST- Posted in
- CPUs
- Cloud Computing
- Amazon
- AWS
- Servers
- Neoverse N1
- Graviton2
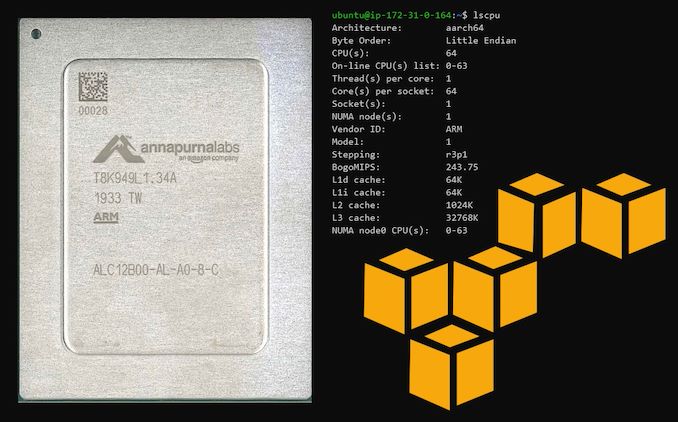
It’s been a year and a half since Amazon released their first-generation Graviton Arm-based processor core, publicly available in AWS EC2 as the so-called 'A1' instances. While the processor didn’t impress all too much in terms of its performance, it was a signal and first step of what’s to come over the next few years.
This year, Amazon is doubling down on its silicon efforts, having announced the new Graviton2 processor last December, and planning public availability on EC2 in the next few months. The latest generation implements Arm’s new Neoverse N1 CPU microarchitecture and mesh interconnect, a combined infrastructure oriented platform that we had detailed a little over a year ago. The platform is a massive jump over previous Arm-based server attempts, and Amazon is aiming for nothing less than a leading competitive position.
Amazon’s endeavours in designing a custom SoC for its cloud services started back in 2015, when the company acquired Isarel-based Annapurna Labs. Annapurna had previously worked on networking-focused Arm SoCs, mostly used in products such as NAS devices. Under Amazon, the team had been tasked with creating a custom Arm server-grade chip, and the new Graviton2 is the first serious attempt at disrupting the space.
So, what is the Graviton2? It’s a 64-core monolithic server chip design, using Arm’s new Neoverse N1 cores (Microarchitectural derivatives of the mobile Cortex-A76 cores) as well as Arm’s CMN-600 mesh interconnect. It’s a pretty straightforward design that is essentially almost identical to Arm’s 64-core reference N1 platform that the company had presented back a year ago. Amazon did diverge a little bit, for example the Graviton2’s CPU cores are clocked in at a bit lower 2.5GHz as well as including only 32MB instead of 64MB of L3 cache into the mesh interconnect. The system is backed by 8-channel DDR-3200 memory controllers, and the SoC supports 64 PCIe4 lanes for I/O. It’s a relatively textbook design implementation of the N1 platform, manufactured on TSMC’s 7nm process node.
The Graviton2’s potential is of course enabled by the new N1 cores. We’ve already seen the Cortex-A76 perform fantastically in last year’s mobile SoCs, and the N1 microarchitecture is expected to bring even better performance and server-grade features, all whilst retaining the power efficiency that’s made Arm so successful in the mobile space. The N1 cores remain very lean and efficient, at a projected ~1.4mm² for a 1MB L2 cache implementation such as on the Graviton2, and sporting excellent power efficiency at around ~1W per core at the 2.5GHz frequency at which Amazon’s new chip arrives at.
Total power consumption of the SoC is something that Amazon wasn’t too willing to disclose in the context of our article – the company is still holding some aspects of the design close to its chest even though we were able to test the new chipset in the cloud. Given the chip’s more conservative clock rate, Arm’s projected figure of around 105W for a 64-core 2.6GHz implementation, and Ampere’s recent disclosure of their 80-core 3GHz N1 server chip coming in at 210W, we estimate that the Graviton2 must come in around anywhere between 80W as a low estimate to around 110W for a pessimistic projection.
Testing In The Cloud With EC2
Given that Amazon’s Graviton2 is a vertically integrated product specifically designed for Amazon’s needs, it makes sense that we test the new chipset in its intended environment (Besides the fact that it’s not available in any other way!). For the last couple of weeks, we’ve had preview access for Amazon Web Services (AWS) Elastic Compute Cloud (EC2) new Graviton2 based “m6g” instances.
For readers unfamiliar with cloud computing, essentially this means we’ve been deploying virtual machines in Amazon’s datacentres, a service for which Amazon has become famous for and which now represents a major share of the company’s revenues, powering some of the biggest internet services on the market.
An important metric determining the capabilities of such instances is their type (essentially dictating what CPU architecture and microarchitecture powers the underlying hardware) and possible subtype; in Amazon’s case this refers to variations of platforms that are designed for specialised use-cases, such as having better compute capabilities or having higher memory capacity capabilities.
For today’s testing we had access to the “m6g” instances which are designed for general purpose workloads. The “6” in the nomenclature designates Amazon’s 6th generation hardware in EC2, with the Graviton2 currently being the only platform holding this designation.
Instance Throughput Is Defined in vCPUs
Beyond the instance type, the most important other metric that defined an instance’s capabilities is its vCPU count. “Virtual CPUs” essentially means your logical CPU cores that’s available to the virtual machine. Amazon offers instances ranging from 1 vCPU to up to 128, with the most common across the most popular platforms coming in sizes of 2, 4, 8, 16, 32, 48, 64, and 96.
The Graviton2 being a single-socket 64-core platform without SMT means that the maximum available vCPU instance size is 64.
However, what this also means, is that we’re quite in a bit of an apples-and-oranges conundrum of a comparison when talking about platforms which do come with SMT. When talking about 64 vCPU instances (“16xlarge” in EC2 lingo), this means that for a Graviton2 instance we’re getting 64 physical cores, while for an AMD or Intel system, we’d be only getting 32 physical cores with SMT. I’m sure there will be readers who will be considering such a comparison “unfair”, however it’s also the positioning that Amazon is out to make in terms of delivered throughput, and most importantly, the equivalent pricing between the different instance types.
Today’s Competition
Today’s article will focus around two main competitors to the Graviton2: AMD EPYC 7571 (Zen1) powered m5a instances, and Intel Xeon Platinum 8259CL (Cascade Lake) powered m5n instances. At the moment of writing, these are the most powerful instances available from the two x86 incumbents, and should provide the most interesting comparison data.
It’s to be noted that we would have loved to be able to include AMD EPYC2 Rome based (c5a/c5ad) instances in this comparison; Amazon had announced they had been working on such deployments last November, but alas the company wasn’t willing to share with us preview access (One reason given was the Rome C-type instances weren’t a good comparison to the Graviton2’s M-type instance, although this really doesn’t make any technical sense). As these instances are getting closer to preview availability, we’ll be working on a separate article to add that important piece of the puzzle of the competitive landscape.
Tested 16xlarge EC2 Instances | |||
m6g | m5a | m5n | |
CPU Platform | Graviton2 | EPYC 7571 | Xeon Platinum 8259CL |
vCPUs | 64 | ||
Cores Per Socket | 64 | 32 | 24 (16 instantiated) |
SMT | - | 2-way | 2-way |
CPU Sockets | 1 | 1 | 2 |
Frequencies | 2.5GHz | 2.5-2.9GHz | 2.9-3.2GHz |
Architecture | Arm v8.2 | x86-64 + AVX2 | x86-64 + AVX512 |
µarchitecture | Neoverse N1 | Zen | Cascade Lake |
L1I Cache | 64KB | 64KB | 32KB |
L1D Cache | 64KB | 32KB | 32KB |
L2 Cache | 1MB | 512KB | 1MB |
L3 Cache | 32MB shared | 8MB shared per 4-core CCX |
35.75MB shared per socket |
Memory Channels | 8x DDR4-3200 | 8x DDR-2666 (2x per NUMA-node) |
6x DDR4-2933 per socket |
NUMA Nodes | 1 | 4 | 2 |
DRAM | 256GB | ||
TDP | Estimated 80-110W? |
180W | 210W per socket |
Price | $2.464 / hour | $2.752 / hour | $3.808 / hour |
Comparing the Graviton2 m6g instances against the AMD m5a and Intel m5n instances, we’re seeing a few differences in the hardware capabilities that power the VMs. Again, the most notorious difference is the fact that the Graviton2 comes with physical core counts matching the deployed vCPU number, whilst the competition counts SMT logical cores as vCPUs as well.
Other aspects when talking about higher-vCPU count instances is the fact that you can receive a VM that spans across several sockets. AMD’s m5a.16xlarge here is still able to deploy the VM on a single socket thanks to the EPYC 7571’s 32 cores, however Intel’s Xeon system here employs two sockets as currently there’s no deployed Intel hardware in EC2 which can offer the required vCPU count in a single socket.
Both the EPYC 7571 and the Xeon Platinum 8259CL are parts which aren’t publicly available or even listed on either company’s SKU list, so these are custom parts for the likes of Amazon for datacentre deployments.
The AMD part is a 32-core Zen1 based single-socket solution (at least for the 16xlarge instances in our testing) clocking in at 2.5 GHz all-cores to up to 2.9GHz in lightly threaded scenarios. The peculiarity of this system is that it’s somewhat limited by AMD’s quad-chip MCM system which has four NUMA nodes (one per chip and 2-channel memory controller), a characteristic that’s been eliminated in the newer EPYC2 Zen2 based systems. We don’t have concrete confirmation on the data, but we suspect this is a 180W part based on the SKU number.
Intel’s Xeon Platinum 8259CL is based on the newer Cascade Lake generation CPU cores. This particular part is also specific to Amazon, and consists of 24 enabled cores per socket. To reach the 16xlarge 64 vCPU count, EC2 provides us a dual-socket system with 16 out of the 24 cores instantiated on each socket. Again, we have no confirmation on the matter, but these parts should be rated at 210W per socket, or 420W total. We do have to remind ourselves that we’re only ever using 66% of the system’s cores in our instance, although we do have access to the full memory bandwidth and caches of the system.
The cache configuration in particular is interesting here as things differ quite a bit between platforms. The private caches of the actual CPUs themselves are relatively self-explanatory, and the Graviton2 here does provide the highest capacity of cache out of the trio, but is otherwise equal to the Xeon platform. If we were to divide the available cache on a per-thread basis, the Graviton2 leads the set at 1.5MB, ahead of the EPYC’s 1.25MB and the Xeon’s 1.05MB. The Graviton2 and Xeon systems have the distinct advantage that their last level caches are shared across the whole socket, while AMD’s L3 is shared only amongst 4-core CCX modules.
The NUMA discrepancies between the systems aren’t that important in parallel processing workloads with actual multiple processes, but it will have an impact on multi-threaded as well as single-threaded performance, and the Graviton2’s unified memory architecture will have an important advantage in a few scenarios.
Finally, there’s quite a difference in the pricing between the instances. At $2.46 per hour, the Graviton2 system edges out the AMD system in price, and is massively cheaper than the $3.80 per hour cost of the Xeon based instance. Although when talking about pricing, we do have to remember that the actual value delivered will also wildly depend on the performance and throughput of the systems, which we’ll be covering in more detail later in the article.
We thank Amazon for providing us with preview access to the m6g Graviton2 instances. Aside from giving us access, Amazon nor any other of the mentioned companies have had influence in our testing methodology, and we paid for our EC2 instance testing time ourselves.
CPU Chip Topologies
Having explained that the various cloud instances can vary quite dramatically in their hardware configurations even though on paper they have the same delivered “vCPU” count, it would be interesting to dwell a little bit more into the CPU topologies and resulting aspects such as the core-to-core latencies. Frustrated with some inflexible or inaccurate public tools on the matter, I recently had the time to write a new custom microbenchmark for testing synchronisation latencies of CPU cores, exhibiting some of the cache-coherency as well as physical layouts of current designs.
The following tables are core-to-core synchronisation latencies in nanoseconds.
Graviton1 - a1.4xlarge - Arm v8.0 ldaex/stlex
I thought that first it would be interesting to go back and showcase how Amazon’s first-generation Graviton SoC fared in this regard. Powered by Cortex-A72 cores, this design had its 16 cores arranged into 4 clusters, connected via coherent crossbar interconnect. Each cluster of 4x A72 cores had its own 2MB L2 cache, and we clearly see the faster access latencies within such a cluster in the above results. Coherency going from one cluster to the other incurred quite a high penalty, almost quadrupling the access latency.
Graviton2 - m6g.16xlarge - Arm v8.0 ldaex/stlex
Cache line Near Core 0
Moving onto the Graviton2 with its 64 N1 cores, we see quite a different setup that is comparatively a lot more uniform. This makes sense as the chip’s cores are connected via a mesh network, and the chip is a single monolithic die design. We do see some odd results in the latencies though, most particularly with the lower numbered cores having seemingly better access latencies between each other than the higher numbered cores. I didn’t quite understand this behaviour as in theory the latencies should behave more evenly across the mesh.
Experimenting around, I saw that the results weren’t consistent across runs. Changing the CPU affinity around did have some larger impact on the results, until I understood what was happening.
Graviton2 - m6g.16xlarge - Arm v8.0 ldaex/stlex
Cache line Near Core 63
In fact, what we’re seeing in the two above result sets isn’t the core-to-core two-point latency across the mesh, but rather the core-to-cache-to-core three-point latencies of the system. Amazon and Arm had confirmed one particular odd aspect of the CMN-600: cache lines are statically resident across the mesh’s cache slices. When allocating a cache line in the memory address space, this undergoes a particular hashing function which determines on which mesh cache slice it is physically homed in. When accessing this cache line from any CPU in the system, it always accesses the same physical L3 cache slice on the chip.
My particular test here consists of three primary threads: the main timing thread, and two ping-pong’ing bouncer threads which alter a flag on a cache-line allocated at the start of the test on the main thread. The behaviour which ensues in the above results table, is that when the flag cache line resides in one corner of the chip (Core pair 54 & 55 to be exact in this results set), and we have two cores on the opposite side of the chip accessing this cache line, it means the data must move forth across the whole chip and back even though it’s only being used by two cores adjacent to each other. Similarly, if two cores are synchronising on a cache line that is homed on a physically near cache slice in the mesh, the latencies will be significantly better as it has to travel much less distance.
It’s quite the odd behaviour that we don’t see quite as prevalent in other meshed cache interconnect systems such as Intel’s Xeons, although admittedly we don’t know if that’s just because we haven’t seen the same large core count implemented in such a manner, or if they have some sort of smarter cache line handling in such scenarios.
Amazon’s Graviton2 doesn’t partition the L3 mesh cache when launching lower vCPU count instances, and each instance in the system competitively shares the full 32MB cache with each other. I didn’t further spend time on this theory in my testing time, but it would be quite the odd result if you’re running some sort of high-synchronisation bottlenecked workload and the performance would vary just depending on where your shared cache line ends up on the chip relative to your active instance cores, food for thought for some database workloads.
The above results were core-to-core latencies implemented via Arm v8.0 exclusive load and stores, which isn’t really how you should do things on new chips such as the Neoverse N1 based Graviton2. As the N1 cores are v8.2 compatible, it means that they also implement the v8.1 ISA which add new instructions such as atomic compare-and-set (CAS).
Graviton2 - m6g.16xlarge - Arm v8.1 CAS
Using CAS instructions for synchronisation is massively more efficient and faster than using several sequential operations for altering a value, as the change can be done in a single atomic operation, vastly reducing the back-and-forth coherency traffic across the chip. In absolute figures, this results in latencies almost 4 times faster than the equivalent Arm v8.0 mechanism.
What’s important here in terms of takeaways, is that if you are deploying software on the new Graviton2 system and upcoming other Neoverse N1 platforms, is that you should pay very close attention to make sure your software stack is compiled against Arm v8.1 or higher (N1 is v8.2).
Currently I don’t know how many pre-compiled packages in Linux distributions even account for such scenarios as I imagine they’re all targeting the most common v8.0 baseline. Again, database applications and other synchronization heavy workloads will be most affected and it’s recommended you compile these from source – it would be an interesting performance test for AWS users who do deploy such workloads (Taking suggestions on such a real-world test setup!).
Looking at the CAS results again, besides the lower latencies, we also see a higher-level latency pattern across the mesh, with distinct separation into 16-core groups in terms of the latencies.
Essentially what I think we’re seeing here is the separation of the chip’s mesh network into “super tiles”, essentially mesh quadrants on the chip. Depending on how the data is routed on the mesh, this appears as a pattern across the latencies between the cores. It is quite odd that these results aren’t as uniform as the v8.0 results; I don’t have any technical explanation for this behaviour.
Finally, it’s again noteworthy that we’re talking about a 64-core system with a single uniform memory domain and on a single monolithic die. AMD’s Rome does provide the former, but doesn’t achieve the same uniform access latencies as the Graviton2.
Xeon Platinum 8259 (Cascade Lake) - m5n.16xlarge - x86 CAS
Looking at the Intel Xeon based instances for comparison, we see relatively uniform access across 16 cores in the same socket, with worse latencies for the CPUs in the second socket. The Graviton2’s latencies here are better than Intel in the best case, but also slightly worse in the worst case (in the same socket).
It’s to be noted that these systems have the SMT logical cores enumerated not at N+1 as in Windows, but instead first listing the cores in physical order first, and then listing the secondary SMT logical cores, hence the mirrored latencies from core 32 onwards. Noteworthy is the low logical-to-logical core latencies of 9.3ns (~3.2GHz cores), due to the coherency between such siblings happening at the L1D cache level.
Having two sockets, we’re seeing two NUMA nodes for the Xeon system, dividing up the physical memory into two virtual memory spaces and two core sets.
AMD EPYC 7571 - m5a.16xlarge - x86 CAS
Finally, the AMD EPYC system we see cores within an CCX having excellent latencies between each other, however coherency between different CCXs is slow, and even slower when accessing other dies within the socket.
The four NUMA nodes means the memory is divided into four virtual memory spaces as well as CPU groups. Again, AMD’s newer EPYC2 Rome processors get rid of this limitation, but alas weren’t able to test on AWS at this moment in time.
Memory Subsystem & Latency
Memory performance in server chips is absolutely crucial due to the sheer core count in the system. Amazon’s Graviton2 chip has the most modern memory capabilities of our test set thanks to 8 DDR4-3200 memory controllers, providing up to a theoretical 204GB/s peak bandwidth. What’s also important, is the SoC’s cache hierarchy and the latencies it’s able to access data at.
Looking at the linear latency graph results, let’s first focus on the DRAM region and see how the Graviton2 ends up relative to the competition.
Surprisingly enough, the Graviton2 does extremely well. Although the cache hierarchies between the designs are very different, when looking at an arbitrary 128MB memory depth, the three systems are near identical. We do see that the Graviton2’s full random latency increases at a higher rate the deeper into DRAM you compare it against the AMD and Intel systems. The structural memory latency between the Amazon and AMD chips are near identical, meaning the AMD system doing better further down in random accesses probably is due to better TLBs or page-table walkers.
Our measured 81ns structural estimate figure here almost directly matches up with Arm’s published 83ns figure from a year ago, further giving credence to Arm’s published figures from back then (Arm's figure was LMBench random using hugepages, we're accounting for TLB misses in our patterns with 4KB pages).
Turning to a logarithmical representation of the same data, we better see the difference in the cache hierarchy.
Compared to the AMD and Intel CPUs, we see the N1 cores’ advantage in the doubled 64KB L1D cache. Access latencies between the different cores should be 4 cycles, with the absolute figures in nanoseconds only differing due to the clock frequency differences between the cores.
The L2 cache of the Graviton2 falls in at 1MB and the access latency here is also competitive at 11 cycles. Arm gives the option between a 512KB 9 cycle or a 1MB 11 cycle configuration, and Amazon’s designers here chose the latter option. Halfway through the 1MB L2 cache we see the latencies of some access patterns increase, and this is due to the test exceeding the capacity of the L1 TLB which falls in at 48 pages (192KB coverage) for the N1 cores, also resulting in the big jump in the TLB miss penalty curve. AMD and Intel here go up to 64 pages and 256KB coverage. To be noted in these results is AMD’s prefetchers pulling into L2, whereas Arm and Intel cores only pull into L3 for more complex patterns.
Going beyond the L2, we reach the L3 where we’re able to test Arm’s CMN-600 mesh interconnect for the first time. The cache hierarchy covers 32MB depth; the interesting aspect here is that the latency remains relatively flat and within 2ns when testing some patterns between 3MB and 32MB, meaning there's fine-grained access hashing across the chip's slices.
The average estimate structural latency of the cache falls in at around 29.6ns, which isn’t all too great when compared to Intel’s ~18.9ns L3 cache, even considering that this is split up across 32 slices versus Intel’s 24 slices. Of course, AMD’s L3 leads here at only 10.6ns, but that’s only shared within 4 CPU cores and doesn’t go nearly as deep.
What we’re also seeing here is that the Graviton2’s N1 cores prefetchers aren’t set up to be nearly as aggressive in some more complex patterns than what we saw in its mobile Cortex-A76 siblings; it’s likely that this was done on purpose to avoid unnecessary memory traffic on the chip, as with 64 cores you’re going to be very bandwidth starved, and you don’t want to waste any of that on possible mis-prefetching.
Moving onto bandwidth testing, we’re solely looking at single-core bandwidth here.
Things are looking massively impressive for the Graviton2’s Neoverse N1 cores as a single CPU core is able to stream writes at up to 36GB/s. What interesting here is that the N1 cores like the Cortex-A76 cores here take advantage of the relaxed memory ordering of the Arm architecture to essentially behave the same as non-temporal writes would on an x86 system, and that’s why the bandwidth if flat across the whole test depth.
Loading from memory achieves up to 18.3GB/s and memory copy (flip test) achieves an impressive 29.57GB/s, which is more than double what’s achieved on the AMD system, and almost triple the Intel system. From a single-core perspective, it seems that the Arm design is able to have significantly better memory capabilities.
We’re still seeing the odd zig-zagging behaviour in the L1 and L2 caches for memory copies that we saw on mobile A76 based chips, possibly cache bank access conflicts for this particular test that showcase in Arm's new microarchitecture.
I didn’t have a proper good multi-core bandwidth test available in my toolset (going to have to write one), so fell back to Timo Bingmann’s PMBW test for some quick numbers on the memory bandwidth scaling of the Graviton2.
The AMD and Intel systems here aren’t quite representative as the test isn’t NUMA aware and that adds a bit of complexity to the matter – as mentioned, we’ll need to write a new custom tool that’s a bit more flexible and robust.
The Arm chip is quite impressive, and we only seemingly needed 8 CPU cores to saturate the write bandwidth of the system, and only 16 cores for the read bandwidth, with the highest figure reaching about 190GB/s, near the theoretical 204GB/s peak of the system, and this is only using scalar 64B accesses. Very impressive.
Compiler Setup, GCC vs LLVM
For further performance testing of the systems, we fell back to SPEC2006 and 2017. I wanted to make sure that there’s no heated discussions when it comes to the compilation of the test suites, so carefully investigated the compilers out there, particularly regarding the choice between GCC and LLVM.
Overall, I checked three different compiler setups: A freshly compiled GCC 9.2.0 release, Arm’s Allinea Studio Compiler 20 package which comes with both Arm’s closed source LLVM and Flang variants as well as a pre-compiled version of GCC 9.2.0, and Marvell’s branch of LLVM and Flang.
We had seen quite a push by Arm for us to consider GCC more closely than LLVM, as Arm had admitted that they’ve spent more time upstream optimising GCC than they’ve had for LLVM. Given the much more prevalent use of GCC in cloud and datacentre applications, I did somewhat agree with this given that’s most likely what you’ll see people use in such environments.
I ran some single-threaded tests across the different compiler setups, the compiler flags were straightforward with just a simple -Ofast
flag as well as -march/-mcpu=cortex-a76
or =neoverse-n1
(alias) for the Arm compiler setup.
As always, our SPEC results aren't officially submitted results, and thus we have to label them merely as "estimates" for this article. Furthermore, SPEC2006 has been retired in favour of SPEC2017, but I still wanted to put up the figures for historical context, as well as mobile comparisons.
Graviton2 SPEC - Single Threaded - 2.5GHz
The overall results favour GCC in the SPECint workloads, while LLVM seemingly does better in the FP and memory heavy tests. Between the upstream GCC 9.2.0 and Arm’s precompiled version there’s seemingly no performance difference whatsoever, while there is some minor difference between Marvell’s setup and Arm’s branch of LLVM.
I ended up going forward with a clean compile of GCC 9.2.0 both for the Arm as well as x86 systems – meaning we’re using the exact same compiler for both architectures, just with different compile targets.
For x86, we’re again using the simple -Ofast
flag for optimisations, and using the corresponding -march/-mtune
targets for the EPYC and Intel platforms, meaning zenver1
and skylake-avx512
.
Overall, it’s a bit odd to see GCC ahead in that many workloads given that LLVM the is the primary compiler for billions of Arm devices in the mobile space. Arm has said that they’re trying to put more effort into this compiler as seemingly it’s lagging behind GCC in terms of some optimisations.
SPEC - Single Threaded Performance
We have some great expectations for the single-threaded performance of the Graviton2 and the Neoverse N1 CPU. In the mobile space, we’ve already seen the Cortex-A76 showcase some extremely competitive performance when compared to x86 platforms running at server frequencies. In particular, the comparison against the first-generation Graviton SoC and its Cortex-A72 cores should be interesting, so I also went ahead and also included comparison numbers on that platform – these figures should put better context into the massive generational uplift that Arm has achieved.
The performance figures tested here are not on a full vCPU instance of the platforms, but rather on “xlarge” variants with only 4 vCPUs, reason for this was simply we didn’t feel too much like paying 95% more for the computing time while the rest of the cores were sitting idle. This isn’t exactly the most optimal method for testing single-threaded performance though, depending on the platform.
One thing to consider in such a small vCPU instance is that you’re only using a fraction of the hardware platform for yourself, while there’s a possibility that there’s other users on other VMs running on the same platform. Such a setup is called having “noisy neighbours”, essentially meaning you’re co-hosted with other users on the same hardware. I did try to verify the figures by running them a few times, and the numbers were consistent on the Graviton2 and AMD platforms. The Graviton2 is still on preview availability so I don’t expect many users using up Amazon’s current deployments, and the AMD unit seemingly didn’t have issues and looked to remain at 2.9GHz throughout most of the testing. On the Intel Xeon platform however, I did see some larger variations, and I think that was mostly due to noisy neighbours brining down the boost clocks of the system down from its 3.2GHz peak. The published numbers here is the higher result set which should be running at around 3.2GHz.
Starting off with SPECint2006, the Graviton2 and N1 CPU are doing extremely well. It’s showcasing almost double the ST performance across the table compared to the A72 based SoC, and it’s even beating the EPYC 7571 across most benchmarks, slightly lagging behind the Xeon instance in some benchmarks.
The Graviton2 is doing particularly well in the memory tests, and latency sensitive tests like 429.mcf are faring significantly better than what we see on the mobile Cortex-A76 SoCs.
In the C/C++ tests of SPECfp2006 (identical set to what se test on mobile, no Fortran compiler available on those platforms), we see the Graviton2 do even better. The delta to the Cortex-A72 platform is even bigger thanks to the more memory sensitive nature of these tests. Here, the Graviton2 is also a lot closer to the x86 competition, staying neck-in-neck with the AMD and Intel platforms.
For the aggregate stores in SPEC2006, the performance uplift compared to the first-gen Graviton is 2x in integer workloads, and 2.2x in FP workloads. Intel is slightly ahead in integer ST performance here, but that gap is reduced to a very thin margin on the FP tests. It’s a great showcase of the Neoverse N1’s IPC capabilities, as the cores are only running at 2.5GHz compared to ~2.9GHz for the AMD system and ~3.2GHz for the Intel system.
Compared to a mobile Cortex-A76 such as in the Kirin 990 (which is the best A76 implementation out there), the resulting IPC is 32% better for the Graviton2 in SPECint2006, and 10% better for SPECfp2006. This goes to show what kind of a massive difference the memory subsystem can have on a system that is otherwise similar in terms of the CPU microarchitecture. We must not forget that the N1 here has the whole 32MB L3 cache available all to itself, even when using a smaller two core vCPU instance.
We’re also covering the SPEC2017 results. In general, the new suite slightly changes up the workloads and, in some cases, increases their complexity, but in SPECint2017, there’s also tests which are laxer compared to their 2006 variants, for example 505.mcf is only using half the memory footprint compared to 429.mcf.
Still, the Graviton2 again here is showcasing some extremely good performance across the board, and is largely mimicking the 2006 results.
The fp2017 results are definitely a more complex set, but again, the Graviton 2 doesn’t have issues keeping up, although this time around it does more often than not lose out to the x86 parts.
In SPECint2017 the Graviton2 is able to showcase a better relative positioning compared to the 2006 tests, just shy of keeping up with the 3.2GHz Cascade Lake system, however in the fp2017 results it’s faring a bit worse than the 2006 system, showcasing a larger margin where it falls behind the competition.
Again, compared to the A1 based Graviton1 instances, the new chip essentially showcases double the single-thread performance, signifying that Arm is now able to compete amongst the big boys in the courtyard.
The results here are a bit shy of what Arm had projected for the N1 platform last year, but the reason for that is that Amazon was quite conservative in terms of the clock frequencies of the Graviton2, as well as only employing 32MB of L3 cache versus the 64MB that Arm had envisioned for a 64-core part. At least on the frequency side, Ampere’s new Altra system running at 3GHz should see scores 20% higher than the figures presented by the Graviton2.
Lastly, let’s again not forget that this isn’t the whole competitive landscape as we don’t have AMD Rome-based instances available to us at this point of time, I’m pretty sure those figures will be a larger leap ahead of the pack presented here.
SPEC - Multi-Core Performance Scaling
I did mention the L3 cache of the Graviton2 was shared amongst all its cores, and we also discovered how only 8-16 cores were able to saturate the memory controllers of the system. To put those aspects into better context, I ran the SPEC suites at rate instance numbers, ranging from 16, 32, 48 and the full 64 cores, and normalised the results relative to the per-thread performance showcased in the rate-1 single-threaded runs.
What this attempts to showcase is the performance scaling of the full SoC across varying loads of the different workload types. Scaling linearly across cores might be easy for some workloads, but for anything that even remotely has some kind of memory pressure should see greater slowdowns given that all the threads are competing for the shared L3 and DRAM resources.
The testing here for all figures were done on a 16xlarge instance with 64 vCPUs to avoid the possibility of noisy neighbours, and give better reliability in the lower core count results.
As expected, we’re seeing a quite wide range of results here, and it’s also a good showcase of which SPEC workloads are memory and cache intensive and which are not. Workloads such as 445.gobmk and 456.hmmer aren’t surprising in their near linear scaling as they don’t have too much cache pressure, and the Graviton2’s 1MB L2 per core is also more than enough for 464.h264ref.
On the other hand, well known memory intensive workloads such as 462.libquantum absolutely crater in terms of per-thread performance. This memory bandwidth demanding workload is fully saturating the bandwidth of the system early on with very few cores, meaning that performance barely increases the more threads and cores we throw at it. Such a scaling more or less is mimicked in other workloads of varying cache and memory pressure.
The most worrying result though is 403.gcc. Code compilation should have been one of the bigger use-cases for a platform such as Graviton2, but the platform is having issues scaling well with core count, undoubtedly a result of higher cache pressure of the system. In a single-thread scenario in the system a core would have access to 33MB L2+L3, but when having 64 cores doing the same thing at once you’d end up with only 1.5MB per core, assuming things are evenly competitively shared.
In SPECfp2006, again, we see the well-known memory intensive workloads such as 433.milc and 470.lbm crater in their per-thread performance the more threads you throw at the system, while other workloads are able to scale near linearly with cores.
In SPECint2017, we see the workload changes I referred to previously on the single-threaded page. The new gcc and mcf tests are actually scaling better than their 2006 counterparts due to actually reduced memory pressure on the new tests. It does beg the question of which variant of the test is actually more representative of most workloads of these types.
Compared to the int2017 suite, the fp2017 suite scales significantly worse for a larger number of workloads. When Ampere last week talked about its Altra processor, and that it was “designed for integer workloads”, that didn't make too much sense other than in the context that the N1 cores are missing wider SIMD execution units. What does make sense though is that the floating-point suite of SPEC is a lot more memory intensive and SoCs like the Graviton2 don’t fare as well at higher loaded core-counts.
It will be interesting to see where the Arm chip designers are heading to in regards to this general memory bottleneck. If your workload isn’t too memory intensive then scaling up to such huge core counts is an easy way to scale performance as well. On the opposite end of the spectrum on memory hungry workloads, these chips will just be memory starved. Arm had envisioned 64 core Neoverse N1 systems to have 64-128MB of L3 cache, and the CMN-600 scales up to 256MB total in a 128-core system, which seem like more sensible and balanced targets.
SPEC - MT Performance (16xlarge 64vCPU)
While the core scaling figures are interesting from an academical standpoint, what’s even more interesting is seeing the absolute throughput numbers compared to the competition. We’re starting off with SPECrate results with 64-rate runs, fully utilising the vCPUs of the EC2 16xlarge instances.
Again, there’s the conundrum of the apples-and-oranges comparison between the Graviton2’s 64 physical cores versus the 32 cores plus SMT setups of the AMD and Intel platforms, but again, that’s how Amazon is positioning these systems in terms of throughput capacity and instance pricing. You could argue that if you can parallelise your workload above a certain amount of threads, it doesn’t matter on whether you can achieve the higher throughput through more cores or through mechanisms such as SMT. Remember, when talking about silicon die area, you could at minimum probably fit 2 N1 cores in the same area than an AMD Zen core or an Intel core (probably an even higher number in the latter comparison).
The Graviton2’s performance is absolutely impressive across the board, beating the Intel Cascade Lake system by quite larger margins in a lot of the workloads. AMD’s Epyc system here doesn’t fare well at all and is showing its age.
It’s particularly in the non-memory bound workloads that the Graviton2 manages to position itself significantly ahead, and here the advantage of having a two-fold physical core lead with essentially double the execution resources shows its benefits.
In the overall SPECrate2006 results, the Graviton2 is shy of Arm’s projection of a 1300 score, but again the Amazon chip does clock in a bit lower and has less cache than what Arm had envisioned in their presentations a year ago.
Nevertheless, the Graviton2 has the performance lead here even against the Intel Cascade Lake based EC2 instances, which is quite surprising given the latter’s cost structure, and indicator of what to come later in the cost analysis.
Arm’s physical core count advantage here continues to show in the execution intensive workloads of SPECint2017, showcasing some very large performance leads in many workloads. The performance leap on important workloads such as 502.gcc again isn’t too great over the Intel system for example – Amazon and Arm definitely could do better here if the chip would have had more cache available.
In SPECfp2017, there’s more workloads in which the Xeon system’s 2-socket setup with a 50% memory channel advantage does show up, able to result in more available bandwidth and thus give the more memory intensive workloads in this suite a good performance advantage over the Graviton2 system. Still, the Arm chip fares very competitively and does put the older AMD EPYC processor in its place, and yes again, we have to remind ourselves that things would be quite different here if we’d be able to include Rome in our charts.
Overall, the Graviton2 system has an undisputed lead in the SPECint2017 suite, whilst just edging out on average the Xeon system in the FP suite, only losing out in situations where the Xeon’s higher memory bandwidth comes at play.
SPEC - MT Performance (4xlarge 16 vCPU)
The 64-core results were quite interesting and put the Graviton2 in a very competitive performance position, but all this talk about performance scaling varying depending on the loaded core count of the system made me wonder how the EC2 instances would perform at lower vCPU counts.
I fired up the same tests, just this time around with only rate-16 to match the number of vCPUs. These are 4xlarge EC2 instances with corresponding 16 vCPUs, but there’s one large caveat in this comparison that we must keep in mind: The Graviton2 instances very likely have no neighbours at this point in time in the test preview, meaning the performance scaling we’re seeing here is very much a best-case scenario for the Amazon chip. EC2 global capacity floats around at 60% active usage, and I imagine Amazon distributes this horizontally across the available sockets in their datacentres. How these performance figures will look like in the real world once Graviton2 ramps up in public availability is anybody’s guess.
The AMD system likely won’t care too much about such scenarios as their NUMA nature means they’re isolated from noisy neighbours anyhow, and we’re just seeing use of a single 8-core chip with its own memory controllers, but the Intel system will have possibly some neighbours doing some activity on the same socket and shared resources. I only ran one test run here; you’d probably need a lot of data to get a representative figure across EC2 usage.
For the Intel m5n instances, using an 4xlarge instance actually means you're only on on single socket this time around, meaning that the scaling behaviour in favour of higher per-thread performance isn't to be expected as high as on the Graviton2 system, as system DRAM bandwidth and L3 is halved compared to the 16xlarge figures on the previous page.
Also, since we’re testing 16 vCPU setups here, we can have an apples-to-apples comparison between the first- and second-generation Graviton systems which should be a fun comparison.
The comparison between the two generations of Graviton processors here is also astounding. Memory intensive workloads favour the newer Graviton2 by at least a factor of 2x, more often 3x, 4x, 5x and even up to 7x in libquantum.
The AMD system as expected doesn’t gain much scaling from using less cores as there’s no more shared resources available on a per-thread basis. The Intel chip fares slightly better per-thread, but doesn’t see the same higher performance scaling (Or should I say, reverse-scaling) as achieved by the Graviton2.
In fp2006, we see more or less the same kind of results.
Overall, in the 16-vCPU rate results the Graviton2 surpasses the performance advantage it showcased in the 64-core results, ending up with an even bigger margin.
The SPEC2017 results again show the same conclusion – the Graviton2 really gains a ton of per-thread performance through the ability to use more of the chip’s L3 cache and 8 memory channels. Whilst on the 64-rate results the Graviton2 and the Xeon were neck-in-neck in fp2017, here the Graviton ends up with a 44% performance advantage.
Again, I can’t put enough emphasis on this, but these results are a best-case scenario for the 4xlarge 16vCPU results of the Graviton2. If production instances are able to achieve such figures will very largely depend on the draw of luck on whether you’re going to be alone on the physical hardware or whether you’ll have any neighbours on the chip. And even if you have neighbours, the performance figures will largely depend on what kind of workloads they will be running alongside your use-cases.
I saw a few articles out there comparing the performance between the m6g instances against the m5 generation instances (Skylake-SP hardware), but most of these tests were done only on medium (1 vCPU) to xlarge (4 vCPUs). When reading such pieces, it’s naturally important to keep in mind the vast scaling advantage the Graviton2 chip has – the smaller your instance is the more chance you’ll have noisy neighbours on the hardware, something that currently just doesn’t happen in the Graviton2’s preview phase.
Cost Analysis - An x86 Massacre
The Graviton2 showcased that it can keep up extremely well in terms of performance and throughput, even beating the competition in a lot of the tests. However sometimes you don’t care too much about performance, and you just want to get some workload completed in the cheapest way possible, at which point value comes into play.
Amazon does allude to that, stating that the new chip is able to achieve 40% better performance per dollar than its competition. As covered in the introduction, for the 64-vCPU count 16xlarge instances the m6g (Graviton2), m5a (EPYC1), and m5n (Xeon Cascade Lake) are priced at an hourly cost of $2.464, $2.752 and $3.808 respectively.
Translating the time to completion of our various SPEC tests to hours and multiplying by the hourly cost, we end up with a cost per fixed workload metric:
An aggregate of all workloads summed up together, which should hopefully end up in a representative figure for a wide variety of real-world use-cases, we do end up seeing the Graviton2 coming in 40% cheaper than the competing platforms, an outstanding figure.
If we were to compare the same fixed workload at smaller instance counts, because of Graviton2’s better per-thread performance, we’re seeing even better results on 4xlarge (16 vCPUs) instances. Here the Amazon chip showcases 43% better value than the Xeon chip, and beats the AMD instances by being 53% cheaper.
If we were to transform the results into a fixed throughput per dollar metric, we again see the Graviton2 far ahead. The unit here is SPEC runs per dollar.
The lower the vCPU instance size, the better value the Graviton2 seemingly becomes, as its performance with increased vCPUs scales sublinearly, but the cost of bigger vCPU instances scales linearly, an effect that’s almost not present at all in the AMD system, and only marginally present in the Xeon instances.
Again, the Graviton2’s scaling here might differ in production instances, but given that you can’t just chop off half the chip (or have access to only one of two sockets, in Intel’s case here) and that Amazon seemingly isn’t doing any static partitioning of the chip’s shared resources, I do think it’s more likely than not that such performance and value figures will be encountered in the real-world.
Even ignoring the lower vCPU instances, Amazon was able to deliver on its promise of 40% better performance per dollar, and it’s a massive shakeup for the AWS and EC2 ecosystem.
Conclusion & End Remarks
We’ve been hearing about Arm in the server space for many years now, with many people claiming “it’s coming”; “it’ll be great”, only for the hype to fizzle out into relative disappointment once the performance of the chips was put under the microscope. Thankfully, this is not the case for the Graviton2: not only were Amazon and Arm able to deliver on all of their promises, but they've also hit it out of the park in terms of value against the incumbent x86 players.
The Graviton2 is the quintessential reference Neoverse N1 platform as envisioned by Arm, aiming for nothing less than disruption of the datacentre market and making Arm servers a competitive reality. The chip is not only able to compete in terms of raw throughput thanks to its 64 physical cores in a single socket, but it also manages to showcase competitive single-thread performance, keeping in line with AMD and Intel systems in the market.
The Amazon chip isn’t perfect, we definitely would have wanted to see more L3 cache integrated into the mesh interconnect as the 32MB does seem quite mediocre for handling 64 cores, and the chip does suffer from this aspect in terms of its performance scaling in memory heavy workloads. Only Amazon knows if this is a real-world bottleneck for the chip and the kind of workloads that are typical in the cloud.
Performance wise, there’s a big empty outline of an elephant in the room that's been missing from our data today, and that’s AMD’s new EPYC2 Rome processors. AMD has showed it had been able to vastly scale performance and do away with a lot of the limitations presented by the first generation EPYC processors that we saw today. Even if we can somewhat estimate the performance that Rome would represent against the Graviton2, we don’t have any idea of what kind of pricing Amazon will be launching the new c5a type instances at.
In terms of value, the Graviton2 seemingly ends up with top grades and puts the competition to shame. This aspect not only will be due to the Graviton2’s performance and efficiency, but also due to the fact that suddenly Amazon is now vertically integrated for its EC2 hardware platforms. If you’re an EC2 customer today, and unless you’re tied to x86 for whatever reason, you’d be stupid not to switch over to Graviton2 instances once they become available, as the cost savings will be significant.
What does this mean for non-Amazon users? Well the Arm server has become a reality, and companies such as Ampere and their new Altra server chips are trying to quickly follow up with the same recipe as the Graviton2 and offer similar ready-made meals for the non-Amazons of the world. These chips however will have to compete with AMD’s Rome, and later in the year the new Milan, which won’t be easy. Meanwhile Intel doesn’t seem to be a likely competitor in the short term while they’re attempting to resolve their issues.
Long-term, things are looking bright for the Arm ecosystem. Arm themselves are aiming to maintain a yearly 20-25% compound annual growth rate for performance, and Ampere already stated they’re looking for yearly hardware refreshes. We don’t know Amazon’s plans, but I imagine it’ll be similar, if not skipping some generations. Around the 2022 timeframe we should see Matterhorn-based products, Arm’s new Very Large™ CPU microarchitecture which should again accelerate things dramatically. In a similar sense, the newly founded Nuvia has lofty goals for their entrance into the datacentre market, and they do have the design talent with a track record to possibly deliver, in a few years’ time.
The Graviton2 is a great product, and we’re looking forward to see more such successful designs from the Arm ecosystem.