
Original Link: https://www.anandtech.com/show/12170/nvidia-titan-v-preview-titanomachy
The NVIDIA Titan V Preview - Titanomachy: War of the Titans
by Ryan Smith & Nate Oh on December 20, 2017 11:30 AM EST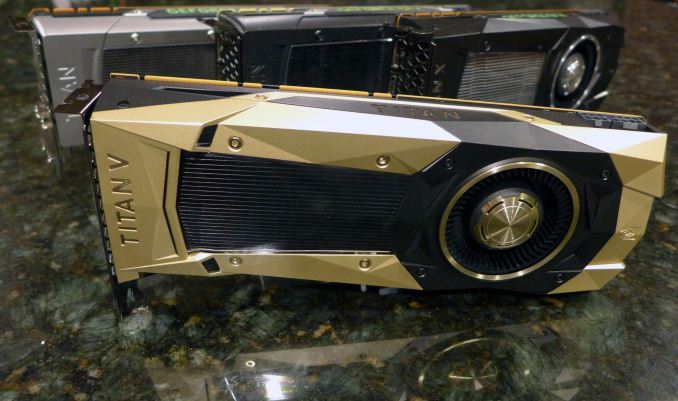
Over the years we at AnandTech have had the interesting experience of covering NVIDIA’s hard-earned but none the less not quite expected meteoric rise under the banner of GPU computing. Nearly a decade ago CEO Jen-Hsun Huang put the company on a course to invest heavily in GPUs as compute accelerators, and while it seemed likely to pay off – the computing industry has a long history of accelerators – when, where, and how ended up being a lot different than Huang was first expecting. Instead of the traditional high performance computing market, the flashpoint for NVIDIA’s rapid growth has been in neural networking, a field that wasn’t even on the radar 10 years ago.
I bring this up because in terms of NVIDIA’s product line, I don’t think there’s a card that better reflects NVIDIA’s achievements and shifts in compute strategy than the Titan family. Though originally rooted as a sort of flagship card of the GeForce family that lived a dual life between graphics and compute, the original GTX Titan and its descendants have instead transitioned over the years into an increasingly compute-centric product. Long having lost its GeForce branding but not the graphical capabilities, the Titan has instead drifted towards becoming a high performance workstation-class compute card. Each generation of the Titan has pushed farther and farther towards compute, and if we’re charting the evolution of the Titan, then NVIDIA’s latest Titan, the NVIDIA Titan V, may very well be its biggest jump yet.
Launched rather unexpectedly just two weeks ago at the 2017 Neural Information Processing Systems conference, the NVIDIA Titan V may be the most important Titan yet for the company. Not just because it’s the newest, or because it’s the fastest – and oh man, is it fast – or even because of the eye-popping $3000 price tag, but because it’s the first card in a new era for the Titan family. What sets the Titan V apart from all of its predecessors is that it marks the first time that NVIDIA has brought one of their modern, high-end compute-centric GPUs to the Titan family, and what that means for developers and users alike. NVIDIA’s massive GV100 GPU, already at the heart of the server-focused Tesla V100, introduced the company’s Volta architecture, and with it some rather significant changes and additions to NVIDIA’s compute capabilities, particularly the new tensor core. And now those features are making their way down into the workstation-class (and aptly named) Titan V.
NVIDIA GPU Specification Comparison | ||||||
Titan V | Titan Xp | GTX Titan X (Maxwell) | GTX Titan | |||
CUDA Cores | 5120 | 3840 | 3072 | 2688 | ||
Tensor Cores | 640 | N/A | N/A | N/A | ||
ROPs | 96 | 96 | 96 | 48 | ||
Core Clock | 1200MHz | 1485MHz | 1000MHz | 837MHz | ||
Boost Clock | 1455MHz | 1582MHz | 1075MHz | 876MHz | ||
Memory Clock | 1.7Gbps HBM2 | 11.4Gbps GDDR5X | 7Gbps GDDR5 | 6Gbps GDDR5 | ||
Memory Bus Width | 3072-bit | 384-bit | 384-bit | 384-bit | ||
Memory Bandwidth | 653GB/sec | 547GB/sec | 336GB/sec | 228GB/sec | ||
VRAM | 12GB | 12GB | 12GB | 6GB | ||
L2 Cache | 4.5MB | 3MB | 3MB | 1.5MB | ||
Single Precision | 13.8 TFLOPS | 12.1 TFLOPS | 6.6 TFLOPS | 4.7 TFLOPS | ||
Double Precision | 6.9 TFLOPS (1/2 rate) |
0.38 TFLOPS (1/32 rate) |
0.2 TFLOPS (1/32 rate) |
1.5 TFLOPS (1/3 rate) |
||
Half Precision | 27.6 TFLOPS (2x rate) |
0.19 TFLOPs (1/64 rate) |
N/A | N/A | ||
Tensor Performance (Deep Learning) |
110 TFLOPS | N/A | N/A | N/A | ||
GPU | GV100 (815mm2) |
GP102 (471mm2) |
GM200 (601mm2) |
GK110 (561mm2) |
||
Transistor Count | 21.1B | 12B | 8B | 7.1B | ||
TDP | 250W | 250W | 250W | 250W | ||
Manufacturing Process | TSMC 12nm FFN | TSMC 16nm FinFET | TSMC 28nm | TSMC 28nm | ||
Architecture | Volta | Pascal | Maxwell 2 | Kepler | ||
Launch Date | 12/07/2017 | 04/07/2017 | 08/02/2016 | 02/21/13 | ||
Price | $2999 | $1299 | $999 | $999 |
Our traditional specification sheet somewhat understates the differences between the Volta architecture GV100 and its predecessors. The Volta architecture itself sports a number of differences from Pascal, some of which we’re just now starting to understand. But the takeaway from all of this is that the Titan V is fast. Tap into its new tensor cores, and it gets a whole lot faster; we’ve measured the card doing nearly 100 TFLOPs. The GV100 GPU was designed to be a compute monster – and at an eye-popping 815mm2, it’s an outright monstrous slab of silicon – making it bigger and faster than any NVIDIA GPU before it.
That GV100 is appearing in a Titan card is extremely notable, and it’s critical to understanding NVIDIA’s positioning and ambitions with the Titan V. NVIDIA’s previous high-end GPU, the Pascal-based GP100, never made it to a Titan card. That role was instead filled by the much more straightforward and consumer-focused GP102 GPU, leading to the resulting Titan Xp. Titan Xp itself was no slouch in compute or graphics, however it left a sizable gap in performance and capabilities between it and the Tesla family of server cards. By putting GV100 into a Titan card, NVIDIA has eliminated this gap. However it also changes the market for the card and its expectations.
The Titan family has already been pushing towards compute for the past few years, and by putting the compute-centric GV100 into the card, NVIDIA has essentially ushered that transition to completion. The Titan V now gets all of the compute capabilities of NVIDIA’s best GPU, but in turn it’s more distant than ever from the graphics world. Which is not to say that it can’t do graphics – as we’ll see in detail in a bit – but this is first and foremost a compute card. In particular it is a means for NVIDIA to seed development for the Volta architecture and its new tensor cores, and to give its user base a cheaper workstation-class alternative for smaller-scale compute projects. The Titan family may have started as a card for prosumers, but the latest Titan V is more professional than any card before.
Putting this into context of what it means for existing Titan customers, and it means different things for compute and graphics customers. Compute customers will be delighted at the performance and the Volta architecture’s new features; though they may be less delighted at the much higher price tag.
Gamers on the other hand are in an interesting bind. Make no mistake, the Titan V is NVIDIA’s fastest gaming card to date, but as we’re going to see in our benchmarks, at least right now it’s not radically ahead of cards like the GeForce GTX 1080 and its Titan Xp equivalent. As a result, you can absolutely game on the card and boutique system builders are even selling gaming systems with the cards. But as we’re going to see in our performance results, the performance gains are erratic and there are a number of driver bugs that need squashed. The end result is that the messaging from NVIDIA and its partners is somewhat inconsistent; the $3000 price tag and GV100 GPU scream compute, but then there’s the fact that it does have video outputs, uses the GeForce driver stay, and is NVIDIA’s fastest GPU to date. I expect interesting things once we have proper consumer-focused Volta GPUs from NVIDIA, but that is a proposition or next year.
Getting down to the business end of things, let’s talk about today’s preview. In Greek mythology Titanomachy was the war of the Titans, and for our first look at the Titan V we’re staging our own version of Titanomachy. We’ve rounded up all four of the major Titans, from the OG GTX Titan to the new Titan V, and have tested them on a cross-section of compute, gaming, and professional visualization tasks in order to see what makes the Titan V tick and how the first graphics-enabled Volta card fares. Today’s preview is just that, a preview – we have even more benchmarks cooking in the background, including some cool deep learning stuff that didn’t make the cut for today’s article. But for now we have enough data pulled together to see how NVIDIA’s newest Titan compares to its siblings, and why the Volta architecture just may be every bit as big of a deal as NVIDIA has been making of it.
The Volta Architecture: In Brief
Before we dive into our benchmark results, I want to spend a bit of time discussing the Volta architecture and the GV100 GPU in particular. Understanding what Volta brings to the table is critical for understanding the performance possibilities of the card, and understanding the GV100 GPU is similarly important for understanding the practical performance of the card.
Volta is a brand new architecture for NVIDIA in almost every sense of the word. While the logical organization is the same much of the time, it's not Pascal at 12nm with Tensor Cores. Rather it's a significantly different architecture in terms of thread execution, thread scheduling, core layout, memory controllers, ISA, and more. And these are just the things NVIDIA is willing to talk about right now, never mind the ample secrets they still keep.
Of the many architectural changes that Volta makes, there are four items in particular that I feel really set it apart from Pascal and help shape its capabilities.
- New tensor cores
- Removing the second warp scheduler dispatch unit & eliminating superscalar execution
- Separating the Integer cores
- Finer-grained thread scheduling
NVIDIA's Big Bet: Tensor Cores
The big story here of course is the new tensor cores. While the Volta architecture has all the makings of a strong HPC architecture even in a traditional context, the specific massive performance numbers that NVIDIA quotes for the Titan V and other Volta cards has come from the use of these tensor cores.
Tensor Cores are a new type of core for Volta that can, at a high level, be thought of as a more rigid, less flexible (but still programmable) core geared specifically for tensor math operations. These cores are essentially a mass collection of ALUs for performing 4x4 Matrix operations; specifically a fused multiply add (A*B+C), multiplying two 4x4 FP16 matrices together, and then adding that result to an FP16 or FP32 4x4 matrix to generate a final 4x4 FP32 matrix. Tensor operations are common in certain types of workloads, but in particular neural networking training and execution (inferencing).
The significance of these cores are that by performing a massive matrix multiplication operation in one unit, NVIDIA can achieve a much higher number of FLOPS for this one operation. A single tensor core performs the equivalent of 64 FMA operations per clock (for 128 FLOPS total), and with GV100 packing 8 such cores per SM, results in 1024 FLOPS per clock per SM. By comparison, even with pure FP16 operations, the standard CUDA cores in a GV100 SM only generate 256 FLOPS per clock. So in scenarios where these cores can be used, we’re looking at the ability to hit 4x the performance versus Pascal.
The flip side to all of this is that the tensor cores are relatively rigid cores. They really aren’t very good for anything except tensor operations, so they are only applicable to certain classes of compute tasks, and right now I don’t know of any graphics tasks that would really benefit from the cores. The benefit to NVIDIA of doing this is that this allows the tensor cores to be very performance dense, both in total performance and in actual die space usage; by lumping together so many ALUs within a single core and without duplicating their control logic or other supporting hardware, the percentage of transistors in a core dedicated to ALUs is higher than on a standard CUDA core. The cost is flexibility, as the hardware to enable flexibility takes up space. So this is a very conscious tradeoff on NVIDIA’s part between flexibility and total throughput.
Meanwhile because tensor cores are brand-new to NVIDIA’s GPUs and because they need to be explicitly called upon, NVIDIA has a bit of a chicken & egg problem here. To make the most of the new hardware, NVIDIA needs developers to write software that taps into the new tensor cores, and in a roundabout way this is one of several roles the Titan V is designed to fill. The $3000 card is still expensive, but it’s the first workstation-class card incorporating these cores, vastly increasing the accessibility of the hardware to software developers. So whereas the first wave of Volta-optimized software has been specific big-ticket items like NVIDIA’s libraries and deep learning frameworks like Caffe2, Titan V will help developers put together the second wave of software.
Scheduling, ILP, & Integers
The second big change that Volta brings to the table is that, at least for GV100, the second warp scheduler dispatch port has been eliminated. Ever since GF104 in 2011, NVIDIA’s architectures have featured two dispatch ports per warp scheduler, allowing for superscalar execution. In other words, their architecture has relied on a degree of instruction level parallelism, requiring the ability to execute a second, non-dependent instruction from a thread in order to get the most out of the hardware.
Volta/GV100, by contrast, is no longer superscalar. Each partition within an SM is now feed by a single dispatch unit warp scheduler, with no opportunity to extract ILP. This means that Volta is a pure thread level parallelism (TLP) design: max utilization comes from maximizing the number of threads active at any given time.
ILP versus TLP is a constant balance, and it’s not unusual to see NVIDIA shifting between the two, especially for a compute-centric GPU like GV100. ILP is nice to have, but extracting it can be difficult. On the other hand while GPUs are meant for embarrassingly parallel tasks, it’s not always easy to generate more threads. So there’s a very real question over whether the performance gains from adding the hardware for ILP justifies the power and complexity costs of doing so.
Meanwhile NVIDIA has also made an interesting change to the Volta architecture with respect to how the integer ALUs are organized. Though not typically a subject of conversation in NVIDIA architecture design, the integer ALUs have traditionally been paired with the FP32 ALUs to make up a single CUDA core. So a black of CUDA cores could either execute integer or floating point operations, but not both at the same time.
Volta in turn separates these ALUs. The integer units have now graduated their own set of dedicates cores within the GPU design, meaning that they can be used alongside the FP32 cores much more freely. The specifics of this arrangement get a bit hairy in light of the fact that Volta isn’t superscalar – so you technically can’t issue INT and FP32 instructions at the same time regardless – but given the fact that most GPU operations take multiple clocks to execute, this allows for much more flexibility than before. NVIDIA notes that it’s especially useful for address generation and in FMA performance, the latter of which we’re taking a look at a bit later.
Finally, and admittedly getting into the even more esoteric aspects of GPU design, NVIDIA has reworked how SIMT works for Volta. The individual CUDA cores within a 32-thread warp now have a limited degree of autonomy; threads can now be synchronized at a fine-grain level, and while the SIMT paradigm is still alive and well, it means greater overall efficiency. Importantly, individual threads can now yield, and then be rescheduled together. This also means that a limited amount of scheduling hardware is back in NV’s GPUs.
This generally doesn’t mean anything for existing software. But for developers who really know their GPUs and threading, it gives them ways to extract performance that couldn’t be done under Pascal’s more rigid SIMT model.
Meet Titan V
Having quickly covered the core architecture, let’s talk about GV100 and the Titan V itself.
Like Pascal before it, for Volta NVIDIA has decided to start big, kicking off the architecture with its flagship compute GPU design, and then letting that cascade down in the future. And NVIDIA didn’t just start big in a metaphorical sense, but in a literal sense as well. At 815mm2 GV100 is massive, even by GPU standards.
This massive GPU comes even after NVIDIA has jumped nodes to TSMC’s highly optimized 16nm FinFET descendant, the aptly named 12nm FFN(vidia) process. At 21.1 billion transistors, NVIDIA has invested all of their gains and then some back into more GPU hardware, pushing the envelope on performance like never before. This is a big part of what makes GV100 such a powerful GPU, though one can only speculate what this is doing for chip yields. Titan V is very clearly a bin for chips that don’t meet the higher requirements of the Tesla V100, and NVIDIA in turn seems to have plenty of Titan V cards available.
By the numbers, GV100 contains 84 SMs. Each SM is, in turn, contains 64 FP32 CUDA cores, 64 INT32 CUDA cores, 32 FP64 CUDA cores, 8 tensor cores, and a significant quantity of cache at various levels. Due to the aforementioned size of the GPU and yield management needs, no product ships with all 84 SMs enabled. Rather both Tesla V100 and Titan V ship with 80 SMs enabled, making for a total of 5120 FP32 CUDA cores and 640 tensor cores.
Like its compute-centric predecessor, GP100, GV100 retains a unique ratio of 64 CUDA cores per SM rather than the usual 128 per SM. This means the ratio of control hardware, cache, and register files to CUDA cores is much higher than on consumer parts. For a compute-centric GPU this makes a lot of sense, however after what NVIDIA did with Pascal and limiting this design to just GP100, it’s worth noting that it’s entirely possible that we’ll see an entirely different SM arrangement on future consumer Volta GPUs.
Also like GP100 before it, GV100’s memory of choice is HBM2. All GV100 packages ship with 4 stacks of the memory, however Titan V only features 3 of those 4 stacks enabled. As this is a salvage part, presumably we’re looking at GV100 packages where there was either a failure in an HBM2 stack, or in the associated memory controller on the GV100 die itself. Either way, this means that Titan V ships with 12GB of VRAM clocked at 1.7Gbps/pin, leading to a total of 653GB/sec of memory bandwidth.
Along with the workstation-suitable card design and inability to use the Tesla driver stack, the memory difference is one of the key differentiators between the Titan V and the PCIe version of the Tesla V100. Otherwise, NVIDIA has confirmed that the Titan V gets the GV100 GPU’s full, unrestricted FP64 compute, FP16 compute, and tensor core performance. To the best of our knowledge (and from what NVIDIA will comment on) it doesn’t appear that they’ve artificially disabled any of the GPU’s core features. So for most use cases, the Titan V is extremely close to the Tesla V100.
In terms of clockspeeds, the HBM2 has been clocked at 1.7GHz, while the 1455MHz boost clock actually matches the 300W SXM2 variant of the Tesla V100, though that accelerator is passively cooled. Notably, the number of tensor cores have not been touched, though the official 110 DL TFLOPS rating is lower than the 1370MHz PCIe Tesla V100, as it would appear that NVIDIA is using a clockspeed lower than their boost clock in these calculations.
For the card itself, it features a vapor chamber cooler with copper heatsink and 16 power phases, all for the 250W TDP that has become standard with the single-GPU Titan models. Output-wise, the Titan V brings 3 DisplayPorts and 1 HDMI connector. And as for card-to-card communication, there is no SLI or NVLink support for the Titan. The PCB itself has NVLink connections on the top, but these have been intentionally blocked by the shroud to prevent their use and are disabled.
Looking at overall performance expectations then, the Titan V is clearly the fastest of the Titans. And yet outside of compute, the advantage for graphics is much smaller. Relative to the Titan Xp we’re looking at just a 14% on-paper advantage in FP32 shader throughput, and thanks to the slightly lower clockspeed an actual ROP throughput disadvantage. The real-world impact of these differences will play out differently among different programs and games, as we’ll see. But it’s an important piece of context all the same. GV100 has a lot of hardware that really only helps compute performance, and from a power standpoint that hardware is a liability. This is why NVIDIA creates differentiated consumer and compute-focused GPUs, and why GV100 isn’t quite as potent for gaming as it may seem.
A Note on Graphics Features
Before diving into our benchmarks, we also wanted to take a quick look at the graphics features of the Titan V. As this is the first Volta card with display outputs, this is our first chance to see if Volta has any new graphics capabilities. NVIDIA for their part has not been discussing Volta’s graphics features in-depth, even with the launch of Titan V, since the focus is on compute.
The flip side to this however is that everything here should still be taken with a grain of salt. Not because it’s inaccurate for Titan V, but because it’s only accurate for GV100 on the current driver stack. This is not a graphics-focused product, and that means there’s no guarantee NVIDIA has every new/upgraded feature exposed. Or for that matter, whether future consumer chips will have identical graphics features.
NVIDIA GPU DirectX Graphics Feature Info | ||||
Volta (Titan V) |
Pascal (Titan Xp) |
|||
Direct3D Feature Level | 12_1 | 12_1 | ||
Fast FP16 Shaders | No | No | ||
Tiled Resources | Tier 3 | Tier 3 | ||
Resource Binding | Tier 3 | Tier 3 | ||
Conservative Rasterization | Tier 3 | Tier 2 | ||
Resource Heap | Tier 1 | Tier 1 |
All of that said, what we find is that indeed, according to NVIDIA’s drivers the graphics capabilities of the Titan V are almost identical to that of the Pascal-based Titan Xp. The latter was already a fairly advanced for its time DirectX feature level 12_1 card, which is still the highest overall feature level tier within DirectX. So any differentiation is limited to the individual features. Which in this case is that the Titan V supports conservative rasterization tier 3 rather than Titan Xp’s more limited tier 2. Outside of software developers this doesn’t mean much at the moment, but it does mean that Volta is the inflection point for when developers can treat conservative rasterization tier 3 as a GPU baseline feature here in half a decade or so.
Meanwhile, as GP100 never came to a card using the GeForce driver set – the closest it got was the Quadro GP100 – this is also our first look at an NVIDIA graphics card with fast FP16 support. A lot has been made of FP16 support in recent years for pixel shaders, as the reduced precision allows for greater shader efficiency and total throughput. The Playstation 4 Pro supports FP16 shaders, as do AMD’s Vega architecture cards.
But for the Titan V, while it has fast FP16 support in hardware, as it turns out this feature hasn’t been exposed to any APIs outside of CUDA. In both Direct3D and OpenGL, FP16 is not exposed and is promoted to FP32 instead. At this point I don’t know of any reason why it needs to be this way – NVIDIA should be able to expose fast FP16 to Direct3D – but for the moment this is not the case. This may be an early driver thing, or if NVIDIA goes the same route with consumer Volta cards as they did Pascal cards, then those cards may not even support fast FP16. In which case there’s little point in enabling fast FP16 support for pixel shaders on the Titan V.
The Test
For gaming, we've opted for 4K-only for this preview, running a subset of our games. Since this is the first Volta card we are benching, we tested both DX11 and DX12 modes for Deus Ex: Mankind Divided and Total War: Warhammer on the Titan V. Load power consumption was measured on Battlefield 1 DX11 on 1440p for the sake of consistency with past results, while average clockspeeds of games were taken at 4K.
And as for our surprise entry at the end, we utilized the venerable Framebuffer Warhead tool, using the 'frost' benchmark with the 64-bit executable. SSAA was enabled in NVIDIA drivers outside the game.
For our preview of the NVIDIA Titan V, we are using NVIDIA’s 388.59 launch driver for all of our Titan cards. Meanwhile, unless explicitly running a FP64 workload, the original GTX Titan was benchmarked with full speed FP64 disabled, as is default for this card.
CPU: | Intel Core i7-7820X @ 4.3GHz |
Motherboard: | Gigabyte X299 AORUS Gaming 7 (BIOS version F7) |
Power Supply: | Corsair AX860i |
Hard Disk: | OCZ Toshiba RD400 (1TB) |
Memory: | G.Skill TridentZ DDR4-3200 4 x 8GB (16-18-18-38) |
Case: | NZXT Phantom 630 Windowed Edition |
Monitor: | LG 27UD68P-B |
Video Cards: | NVIDIA Titan V NVIDIA Titan Xp NVIDIA GeForce GTX Titan X (Maxwell) NVIDIA GeForce GTX Titan |
Video Drivers: | NVIDIA Release 388.59 |
OS: | Windows 10 Pro (Creators Update) |
Compute Performance
Diving in, I want to start with a look at compute performance. Titan V is first and foremost a compute product, so what makes or breaks the card – what justifies its existence – is how well it does at compute.
This is actually a more challenging aspect to test than it may first appear, as NVIDIA’s top-down architecture launch strategy means that there’s not a lot of commercial or otherwise widely-used software written that can take full advantage of the Volta architecture. Even in the major deep learning frameworks, their support for Volta is still in the early phases or still under active development. So I’ve opted to excise the deep learning tests for the moment, while we get a better handle on those for our full review. Meanwhile software that can take advantage of Volta’s fast FP16 and FP64 modes is also quite rare, especially as fast FP16 is not a consumer NVIDIA feature at this time.
Compute overall is harder to test, as unlike games or even professional visualization tasks, there’s far fewer standard workloads. Even tasks using major compute frameworks are often heavily tailored towards a specific need. So while our compute benchmarks are meant to be enlightening, software developers and compute users will want to carefully study how their workloads map to GPU hardware.
Anyhow, before we get too deep here, I want to take a quick look at FMA latency. Over successive generations of GPU architectures NVIDIA has continued to find ways to reduce the latency of what’s perhaps the most critical instruction in the GPU space, and Volta continues this trend.
With Volta NVIDIA has been able to knock another two clock cycles off of the latency for an FMA, reducing it from 6 cycles on Pascal/Maxwell to 4 cycles. This is thanks to NVIDIA’s separating the INT32 and FP32 units in the Volta architecture, which allows more of the overall FMA operation to be performed in parallel. The end result is that for any instructions that depend on the result of an FMA operation, they can kick off 33% sooner, improving overall compute/shader efficiency.
Interestingly this was one area of compute where NVIDIA was trailing AMD – who has an average latency of around 4.5 cycles – so it’s great to see NVIDIA close the gap here. And this shouldn’t be considered a trivial change, as reducing the number of clock cycles for any fundamental operation is quite difficult. Case in point, I’m not sure just how NVIDIA could get FMAs any lower than 4 cycles.
General Matrix Multiply (GEMM) Performance
Starting things off, let’s take a look at General Matrix Multiply (GEMM) performance. GEMM measures the performance of dense matrix multiplication, which is an important operation in a variety of scientific fields. GEMM is highly parallel and typically compute heavy, and one of the first tests of performance and efficiency on any parallel architecture geared towards HPC workloads.
Since matrix multiplication is such a fundamental operation, it’s one of the operations included in NVIIDA’s CUBLAS library, meaning NVIDIA offers a highly optimized version of the function. Importantly, it also means that NVIDIA offers Volta-optimized implementations of the function, including operations that run on the densest matrix multiplication core of them all, the tensor core. So GEMM provides us a fantastic opportunity to look at the performance of not only the usual FP16/FP32/FP64 operations, but also the FP16 tensor cores themselves. This is very much an ideal case, but it gives us the means to see what kind of maximum throughput Titan V can reach.
Burying the lede here, let’s start with single precision (FP32) performance. As mentioned briefly in our look at the specifications of the Titan V, the Titan V is not immensely more powerful than the Titan Xp when it comes to standard FP32 operations. It has a much greater number of FP32 CUDA cores, but the compute-centric chip trades that off with lower average clockspeeds, which dulls the difference some.
None the less, the Titan V is 23% faster in FP32 GEMM, which is actually a greater improvement than the 14% increase that we’d expect going by the specifications. In fact at 13.4 TFLOPS, the Titan V is at 97% of its rated 13.8 TFLOPS FP32 performance, an amazingly efficient metric. The Titan Xp isn’t as efficient here – hitting 10.9 of 12.1 TFLOPs for a 90% rating – underscoring the important architectural improvements Volta brings. I am not 100% sure on just which factors lead to this improvement, but the separation of INT32 and FP32 cores along with the scheduling changes are likely candidates. Titan V also benefits from improvements in caching and more memory bandwidth, which further reduces any potential bottlenecks in feeding the beast.
As for double precision (FP64) and half precision (FP16) performance, these are in line with our expectations. The official FP64 and FP16 rates for Titan V are 1:2 and 2:1 FP32 respectively, and this is what we find on GEMM. Compared to the Titan Xp, which didn’t offer fast FP64 or FP16 modes, the difference is night and day: Titan V is 16x and 121x faster respectively.
Last but not least, let’s talk tensors. I almost removed the tensor core results from this graph, but ultimately I left them in to highlight just what the tensor cores mean for compute developers who have tasks well-suited for them. When configured to use the tensor cores, our GEMM benchmark runs hit 97.5 TFLOPs, 3.7x faster than Titan V’s already fast FP16 performance. Or to put this another way, this one card is doing 97 trillion floating point operations in a single second.
Relative to the high efficiency marks we saw earlier with the CUDA cores, the tensor cores don’t come quite as close. 97.5 TFLOPs is about 89% of the Titan V’s rated 110 TFLOPs tensor core performance. Besides the fact that the tensor cores are extremely dense – they’ll give your power and cooling a good run for their money – I suspect that Titan V is feeling the pinch of memory bandwidth. The loss of a stack of HBM2 means that it’s trying to perform trillions of operations on only billions of bytes of memory bandwidth.
Finally, I can’t reiterate enough that when looking at tensor core performance, context is king here. The tensor cores are very fast, but they are highly specialized. GPUs in general are only good for a subset of overall computing tasks, and the tensor cores take that one step further. In super dense matrix multiplication scenarios they excel, but they have little to offer outside of that. Thankfully for NVIDIA and its newly found deep learning empire, neural networks map very well to tensor operations.
SiSoftware Sandra
Moving on from our low-level GEMM benchmark, let’s move up a level to something a bit more practical with some of SiSoftware’s Sandra’s GPU compute benchmarks. Unfortunately for reasons I haven’t yet been able to determine, Sandra’s CUDA benchmarks aren’t working with NVIDIA’s latest drivers, which limits us to working with OpenCL and DirectX compute shaders. Still, this gives us a chance a look at performance outside of specialized CUDA applications.
Relatively speaking, Titan V once again punches above its weight. In FP32 mode Sandra’s compute shader benchmark clocks the card as processing 25 Gigapixels per second, versus 18.4 for the Titan Xp, a 37% difference and once again exceeding the on-paper differences between these products. I suggest caution in reading too much into these synthetic compute results, as real-world workloads aren’t nearly as neat and tidy. But it continues to point to the Volta architecture having improved things by quite a bit under the hood.
Meanwhile, because NVIDA doesn’t expose fast FP16 mode outside of CUDA, the Titan V’s FP16 performance doesn’t get used to its fullest extent here. Fast FP64 on the other hand is exposed, and Titan V leaves every other Titan in the dust.
The story is much the same using DX11 video (pixel) shaders. Though it’s very interesting that Titan V isn’t showing the same kind of sizable performance gains here as it did using a compute shader.
A bit more real-world is Sandra’s financial analysis benchmark, which performs a series of operations including the ever-important Monte Carlo simulation. Titan V is once again well in the lead here, punching above its weight relative to the Titan Xp. FP64 performance is also much stronger than the other Titans, though on a relative basis, the performance drop-off for moving to FP64 is much more than the 50% performance hit that we’d expect on paper.
Compute Performance: Geekbench 4
In the most recent version of its cross-platform Geekbench benchmark suite, Primate Labs added CUDA and OpenCL GPU benchmarks. This isn’t normally a test we turn to for GPUs, but for the Titan V launch it offers us another perspective on performance.
The results here are interesting. We’re not the only site to run Geekbench 4, and I’ve seen other sites with much different scores. But as we haven’t used this benchmark in great depth before, I’m hesitant to read too much into it. What it does show us, at any rate, is that the Titan V is well ahead of the Titan Xp here, more than doubling the latter’s score.
NVIDIA Titan Cards GeekBench 4 Subscores | ||||||
Titan V | Titan Xp | GTX Titan X | GTX Titan | |||
Sobel (GigaPixels per second) |
35.1 | 24.9 | 16.5 | 9.4 | ||
Histogram Equalization (GigaPixels per second) |
21.2 | 9.43 | 5.58 | 4.27 | ||
SFFT (GFLOPS) |
180 | 136.5 | 83 | 60.3 | ||
Gaussian Blur (GigaPixels per second) |
23.9 | 2.67 | 1.57 | 1.45 | ||
Face Detection (Msubwindows per second) |
21.7 | 12.4 | 8.66 | 4.92 | ||
RAW (GigaPixels per second) |
18.2 | 10.8 | 5.63 | 4.12 | ||
Depth of Field (GigaPixels per second) |
3.31 | 2.74 | 1.35 | 0.72 | ||
Particle Physics (FPS) |
83885 | 30344 | 18725 | 18178 |
Looking at the subscores, the Titan V handily outperforms the Titan Xp on all of the subtests. However it’s one test in particular that stands out here, and is likely responsible for the huge jump in the overall score, and that’s the Gaussian Blur, where the Titan V is 9x (!) faster than the Titan Xp. I am honestly not convinced that this isn’t a driver or benchmark bug of some sort, but it may very well be that Primate Labs has hit on a specific workload or scenario that sees some rather extreme benefits from the Volta architecture.
Folding @ Home
Up next we have the official Folding @ Home benchmark. Folding @ Home is the popular Stanford-backed research and distributed computing initiative that has work distributed to millions of volunteer computers over the internet, each of which is responsible for a tiny slice of a protein folding simulation. FAHBench can test both single precision and double precision floating point performance, giving us a good opportunity to let Titan V flex its FP64 muscles.
A CUDA-backed benchmark, this is the first sign that Titan V’s performance lead over the Titan Xp won’t be consistent. And more specifically that existing software and possibly even NVIDIA’s drivers aren’t well-tuned to take advantage of the Volta architecture just yet.
In this case the Titan V actually loses to the Titan Xp ever so slightly. The scores are close enough that this is within the usual 3% margin of error, which is to say that it’s a wash overall. But it goes to show that Titan V isn’t going to be an immediate win everywhere for existing software.
CompuBench
Our final set of compute benchmarks is another member of our standard compute benchmark suite: CompuBench 2.0, the latest iteration of Kishonti's GPU compute benchmark suite. CompuBench offers a wide array of different practical compute workloads, and we’ve decided to focus on level set segmentation, optical flow modeling, and N-Body physics simulations.
It’s interesting how the results here are all over the place. The Titan V shows a massive performance improvement in both N-Body simulations and Optical Flow, once again leading to the Titan V punching well above its weight. But then the Level Set Segmentation benchmark is practically tied with the Titan Xp. Suffice it to say that this puts the Titan V in a great light, and conversely makes one wonder how the Titan Xp was (apparently) so inefficient. The flip side is that it’s going to be a while until we fully understand why certain workloads seem to benefit more from Volta than other workloads.
Synthetic Graphics Performance
Moving on, let’s take a look at the synthetic graphics performance of the Titan V. As this is the first graphics-enabled product using the GV100 GPU, this can potentially give us some insight into the yet-untapped graphics capabilities of the Volta architecture. However the usual disclaimer about earlier drivers and early hardware is very much in effect here: we don’t know for sure how consumer Volta parts will be configured, and in the meantime it’s not clear just how well the Volta drivers have been optimized for graphics.
Right now the graphics aspects of the Volta architecture are something of a black box, and tessellation performance with TessMark reflects this. On Pascal, NVIDIA has one Polymorph engine per TPC; if this is the same on GV100, then Titan V has around 40% more geometry hardware than Titan Xp. On the other hand, the actual throughput rate of Polymorph engines has varied over the years as NVIDIA has dialed it up or down in line with their performance goals and the number of such hardware units available. So for all we know, Titan V’s Polymorph engines don’t pack the same punch as Titan Xp’s.
At any rate, Titan V once again comes away with a win. But the 16% lead is not a day-and-night difference, unlike what we’ve seen with compute.
Beyond3D Suite
Meanwhile for looking at texel and pixel fillrate, we have the Beyond3D Test Suite.
Starting with the pixel throughput benchmark, I’m actually a bit surprised the difference is as great as it is. As discussed earlier, on paper the Titan V has lower ROP throughput than the Titan Xp, owing to lower clockspeeds. And yet in this case it’s still in the lead by 18%. It’s not a massive difference, but it means the picture is more complex than naïve interpretation of GV100 being a bigger Pascal. This may be an under-the-hood efficiency improvement (such as compression), or it could just be due to Titan V’s raw bandwidth advantage. This will be worth keeping an eye on once NVIDIA starts talking about consumer Volta cards.
As for texel throughput, it’s interesting that INT8 and FP32 texture throughput gains are so divergent. Titan V is showing much greater INT8 texture throughput gains than it is FP32; and Titan Xp was no slouch in that department to begin with. I’m curious whether this is a product of separating the integer ALUs from the FP ALUs on Volta, or whether NVIDIA has made some significant changes to their texture mapping units.
3DMark Fire Strike Ultra
I’ve also gone ahead and run Futuremark’s 3DMark Fire Strike Ultra benchmark on the Titans. This benchmark is a bit older, but it’s a better understood (and better optimized) DX11 benchmark.
The results here find the Titan V in the lead, but not by much: just 8%. Relative to Pascal/Titan Xp, Titan V’s performance advantages and architectural improvements are inconsistent, in as much as not everything has been improved to the same degree. These results point to a bottleneck elsewhere – perhaps the ROPs? – but don’t isolate it. More significantly though, it’s an indication that while Titan V can do graphics, perhaps it’s not well-built (or at least well-optimized) for the task.
SPECViewPerf 12.1.1
Finally, while SPECViewPerf’s component software packages are more for the professional visualization side of the market – and NVIDIA doesn’t optimize the GeForce driver stack for these programs – I wanted to quickly run all of the Titans through the test all the same, just to see what we’d get.
NVIDIA Titan Cards SPECviewperf 12.1 FPS Scores | ||||||
Titan V | Titan Xp | GTX Titan X | GTX Titan | |||
3dsmax-05 | 176.3 | 173.7 | 133.1 | 100.3 | ||
catia-04 | 180.3 | 181.4 | 71.3 | 24.1 | ||
creo-01 | 119.4 | 122.1 | 41.0 | 30.1 | ||
energy-01 | 27.7 | 21.2 | 8.7 | 5.8 | ||
maya-04 | 159.0 | 152.0 | 131.6 | 98.6 | ||
medical-01 | 89.4 | 91.9 | 39.9 | 27.7 | ||
showcase-01 | 175.5 | 167.0 | 98.7 | 67.0 | ||
snx-02 | 223.2 | 225.3 | 7.37 | 4.1 | ||
sw-03 | 110.1 | 100.7 | 52.2 | 41.3 |
The end result is actually quite interesting. The Titan V only wins a bit more than it loses, as the card doesn’t pick up much in the way of performance versus the Titan Xp. Professional visualization tasks tend to be more ROP-bound, so this may be a consequence of that, and I wouldn’t read too much into this versus anything Quadro (or what a Quadro GV100 card could do). But it illustrates once again how Titan V has improved at some tasks by more than it has in others.
Gaming Performance
Sure, compute is useful. But be honest: you came here for the 4K gaming benchmarks, right?
Already after Battlefield 1 (DX11) and Ashes (DX12), we can see that Titan V is not a monster gaming card, though it still is faster than Titan Xp. This is not unexpected, as Titan V's focus is quite far away from gaming as opposed to the focus of the previous Titan cards.
Despite being generally ahead of Titan Xp, it's clear Titan V is suffering from lack of gaming optimization. And for that matter, the launch drivers definitely have bugs in them as far as gaming is concerned. Titan V on Deus Ex resulted in small black box artifacts during the benchmark; Ghost Recon Wildlands experienced sporadic but persistant hitching, and Ashes occasionally suffered from fullscreen flickering.
And despite the impressive 3-digit FPS in the Vulkan-powered DOOM, the card actually falls behind Titan Xp in 99th percentile framerates. For such high average framerates, even a 67fps 99th percentile can reduce perceived smoothness. Meanwhile, running Titan V under DX12 for Deus Ex and Total War: Warhammer resulted in less performance. But with immature gaming drivers, it is too early to say if these are representative of low-level API performance on Volta itself.
Overall, the Titan V averages out to around 15% faster than the Titan Xp, excluding 99th percentiles, but with the aforementioned caveats. Titan V's high average FPS in DOOM and Deus Ex are somewhat marred by stagnant 99th percentiles and minor but noticable artifacting, respectively.
So as a pure gaming card, our preview results indicate that this would not the best gaming purchase at $3000. Typically, a $1800 premium for around 10 - 20% faster gaming over the Titan Xp wouldn't be enticing, but it seems there are always some who insist.
But Can It Run Crysis?
Even if the Titan V isn't a major leap in gaming performance, we couldn't help ourselves. We have a Titan, we have Crysis. The ultimate question must be answered. Can it run Crysis?
Yes, it can run Crysis.
And in fact, it is the only Titan that can reach the coveted 60fps mark. Perhaps Titan V is the card that can finally run Crysis the way it's meant to be played: maximum resolution, maximum details, and maximum anti-aliasing. At the end of the day, only one Titan stands above the rest when it comes to Crytek's testament to graphical intensity.
Power, Temperature, & Noise
Finally, let's talk about power, temperature, and noise. At a high level, the Titan V should not be substantially different from other high-end NVIDIA cards. It has the same 250W TDP, and the cooler is nearly identical to NVIDIA’s other vapor chamber cooler designs. In short, NVIDIA has carved out a specific niche on power consumption that the Titan V should fall nicely into.
Unfortunately, no utilities seem to be reporting voltage or HBM temperature of Titan V at this time. These would be particularly of interest considering that Volta is fabbed on TSMC's bespoke 12FFN as opposed to 16nm FinFET. This also marks the first time NVIDIA has implemented HBM2 in gaming use-cases, where HBM temperatures or voltages could be elucidating.
NVIDIA Titan V and Xp Average Clockspeeds | |||
NVIDIA Titan V | NVIDIA Titan Xp | Percent Difference | |
Idle | 135MHz | 139MHz | - |
Boost Clocks |
1455MHz
|
1582MHz
|
-8.0% |
Max Observed Boost |
1785MHz
|
1911MHz
|
-6.6% |
LuxMark Max Boost | 1355MHz | 1911MHz | -29.0% |
Battlefield 1 |
1651MHz
|
1767MHz
|
-6.6% |
Ashes: Escalation |
1563MHz
|
1724MHz
|
-9.3% |
DOOM |
1561MHz
|
1751MHz
|
-10.9% |
Ghost Recon |
1699MHz
|
1808MHz
|
-6.0% |
Deus Ex (DX11) |
1576MHz
|
1785MHz
|
-11.7% |
GTA V |
1674MHz
|
1805MHz
|
-7.3% |
Total War (DX11) | 1621MHz | 1759MHz | -7.8% |
FurMark |
1200MHz
|
1404MHz
|
-14.5% |
Interestingly, LuxMark only brings Titan V to 1355MHz instead of its maximum boost clock, a behavior that differs from every other card we've benched in recent memory. Other compute and gaming tasks do bring the clocks higher, with a reported peak of 1785MHz.
The other takeaway is that Titan V is consistently outclocked by Titan Xp. In terms of gaming, Volta's performance gains do not seem to be coming from clockspeed improvements, unlike the bulk of Pascal's performance improvement over Maxwell.
Meanwhile it's worth noting that the HBM2 memory on the Titan V has only one observed clock state: 850MHz. This never deviates, even during FurMark as well as extended compute or gameplay. For the other consumer/prosumer graphics cards with HBM2, AMD's Vega cards downclock HBM2 in high temperature situations like FurMark, and also features a low-power 167MHz idle state.
Measuring power from the wall, Titan V's high idle and lower load readings jump out.
Meanwhile under load, the Titan V's power consumption at the wall is slightly but consistently lower than the Titan Xp's. Again despite the fact that both cards have the same TDPs, and NVIDIA's figures tend to be pretty consistent here since Maxwell implemented better power management.
During the course of benchmarking, GPU-Z reported a significant amount of Titan V thermal throttling, and that continued in Battlefield 1, where it oscillated between being capped out by GPU underutilization and temperature. And in FurMark, the Titan V was consistently temperature-limited.
Without HBM2 voltages, it is hard to say if the constant 850MHz clocks are related to Titan V's higher idle system draw. 815mm2 is quite large, but then again elements like Volta's tensor cores are not being utilized in gaming. In Battlefield 1, system power draw is actually lower than Titan Xp but GPU Z would suggest that thermal limits are the cause. Typically what we've seen with other NVIDIA 250W TDP cards is that they hit their TDP limits more often than they hit their temperature limits. So this is an unusual development.
Featuring an improved cooler, Titan V essentially manages the same noise metrics as its Titan siblings.
First Thoughts
Given what we’ve seen over the past half-decade or so with NVIDIA’s success in the GPU computing space, it’s easy to see why NVIDIA has made the architectural decisions that have shaped the Pascal and now Volta architectures. At the same time however, the increasing specialization of NVIDIA’s compute-centric GPUs and resulting products has meant that the company has needed to rethink and refocus the Titan family of video cards. Though originally created for a mixed prosumer market, Titan X and later have seen increasing success not as graphics cards but as workstation-class compute cards as professional customers have invested heavily in deep learning technologies. As a result the Titan line itself has been drifting more and more towards being a compute product, and with the launch of the Titan V, NVIDIA’s latest card takes its biggest step yet in this regard.
By switching the Titan family over from NVIDIA’s consumer GPUs to their compute-centric GV100 GPU, NVIDIA has centered the Titan V on compute more strongly than any Titan before it. And in compute it flies. Though we’ve still only had a chance to scratch the surface of Titan V, GV100, and the Volta architecture in today’s preview, all of our early benchmark data points to something that datacenter customers have already known for several months now: GV100’s not just a bigger NVIDIA GPU, and Volta’s not a minor iteration on Pascal. Sure, GV100 is also literally huge, but under the hood Volta brings a great deal of new architectural improvements and optimizations that, until the launch of the Titan V (and the opportunity to get our hands on the card) we haven’t really had the chance to properly appreciate.
The biggest factor here for NVIDIA is of course the tensor cores. NVIDIA has bet big on what’s essentially a highly specialized dense matrix multiplier, and because it maps so well to the needs of neural network training and execution, this is a bet that appears to already be paying off in spades for the company. As we saw in our GEMM results, if you can really put the tensor cores to good use, then the sheer mathematical throughput is nothing short of immense. But – and this is key – developers need to be able write their software around those tensor cores to get the most out of the Titan V. And I’m unsure whether any of this will translate into the consumer space and use cases, or if this is a feature that’s ultimately going to be mostly adopted by the HPC crown.
However even disregarding the tensor cores, what we’re seeing with the Titan V is that in terms of compute efficiency, this card appears to be on a very different level from the Pascal-based Titan Xp, and that card was no slouch either. I feel like there’s a bit of an apples-to-oranges comparison here since under the hood the cards are so different – the consumer GP102 GPU versus the compute GV100 GPU – but they are still very much Titans. Titan V is punching above its weight in many compute tasks compared to what it should be able to do on paper, and this is thanks in large part to the efficiency gains we’re seeing. For our full review hopefully we can track down a Quadro GP100 and try to better isolate how much of Volta and GV100’s gains come from improvements versus the Pascal architecture, and how much is from the unique, compute-centric configurations of NVIDIA’s Gx100-class GPUs.
For compute customers then, the flip side to all of this is that at $3000, the Titan V is quite the expensive card. Much more so than the mere $1200 of the Titan Xp. As the Titan V doesn’t experience dramatic performance gains in every application, I suspect that Titan compute customers are going to continue picking up both cards for some time to come. But a crazy as a $3000 price tag is, for customers that need to maximize single-GPU performance and/or get their hands on a product with tensor cores, then the Titan V is certainly going to fill this role well. Titan V can absolutely deliver the kind of performance to justify its price in the compute space.
However for graphics customers, we’re looking at a different story. To preface this, I don’t want to entirely discount the Titan V as a video card here, because at the end of the day it’s the fastest video card released by NVIDIA to date, gaming and all. But the immense performance gains we saw in our compute benchmarks – the kind of gains that justify a $3000 price tag – are not present in our gaming benchmarks. Right now the Titan V is moderately faster than the Titan Xp in gaming workloads – and in a world where multi-GPU scaling is on the rocks, that’s a huge element in favor of the Titan V – but that’s counterbalanced by the fact that in some cases the Titan V can’t even beat the Titan Xp.
Now how much of that is because of GV100’s suitability for gaming or how much of it is due to drivers is something that remains to be seen. The Titan V is a new card, the first graphics card based on the Volta architecture, and it’s not at all clear whether NVIDIA’s launch drivers are fully optimized for the new architecture. What is obvious is that the current drivers do have some notable bugs, and that does lend credit to the idea that the launch drivers aren’t fully baked. But even if that’s the case, that’s likely not something worth betting on. The Titan V may use the GeForce driver stack, but mentally it’s better compartmentalized as a compute card that can do graphics on the side, rather than the jack-of-all-trades nature that has previously defined the Titan family.
Closing out today’s preview, it’s hard not to come away impressed with what we’ve seen. But we’ve also just scratched the surface over the span of a few days. We’re going to continue looking at the Titan V, including getting some deep learning software up and running. Which is not to say that Titan V is merely a deep learning accelerator – it’s clearly much more than that – but this is definitely one of the most practical uses for the hardware right now, especially as software developers just now get their hands on Volta. Though for that matter I think it’ll be interesting to see what academics and researchers do with the hardware; they were one of the driving forces behind the original GTX Titan, and it’s no mistake that NVIDIA has a history of giving away Titans to these groups. Part of the need for a card like the Titan V in NVIDIA’s lineup is to help seed Volta in this fashion, so the launch of the Titan V is part of a much larger and longer-term plan by NVIDIA.